Nielsen Norman Group • Tarun Mugunthan and Sarah Gibbons • June 14, 2024
Summary: AI image generation users often follow a similar creative process: ideate, generate, refine, and export.
Understanding how expert users use AI tools in the process of image generation can help designers and other beginner users get better at using these tools for their projects; it can also inform the future design of such tools.
In This Article:
- Our Research
- AI Image-Generation Process: 4 Stages
- Stage 1: Define
- Stage 2: Explore
- Stage 3: Refine
- Stage 4: Export
- Nonlinear Variations
- Conclusion
Our Research
We conducted nine contextual inquiry sessions to understand the users’ natural behaviors when generating images with AI. Participants were invited to use any image generation tool they preferred; all nine chose Midjourney.
During the sessions, we observed patterns in participants’ creative process, regardless of what they were producing. We distilled these user journeys into a high-level experience map to represent how most users approached their goals.
AI Image-Generation Process: 4 Stages
Study participants went through four stages as they generated AI images:
- Define
- Explore
- Refine
- Export
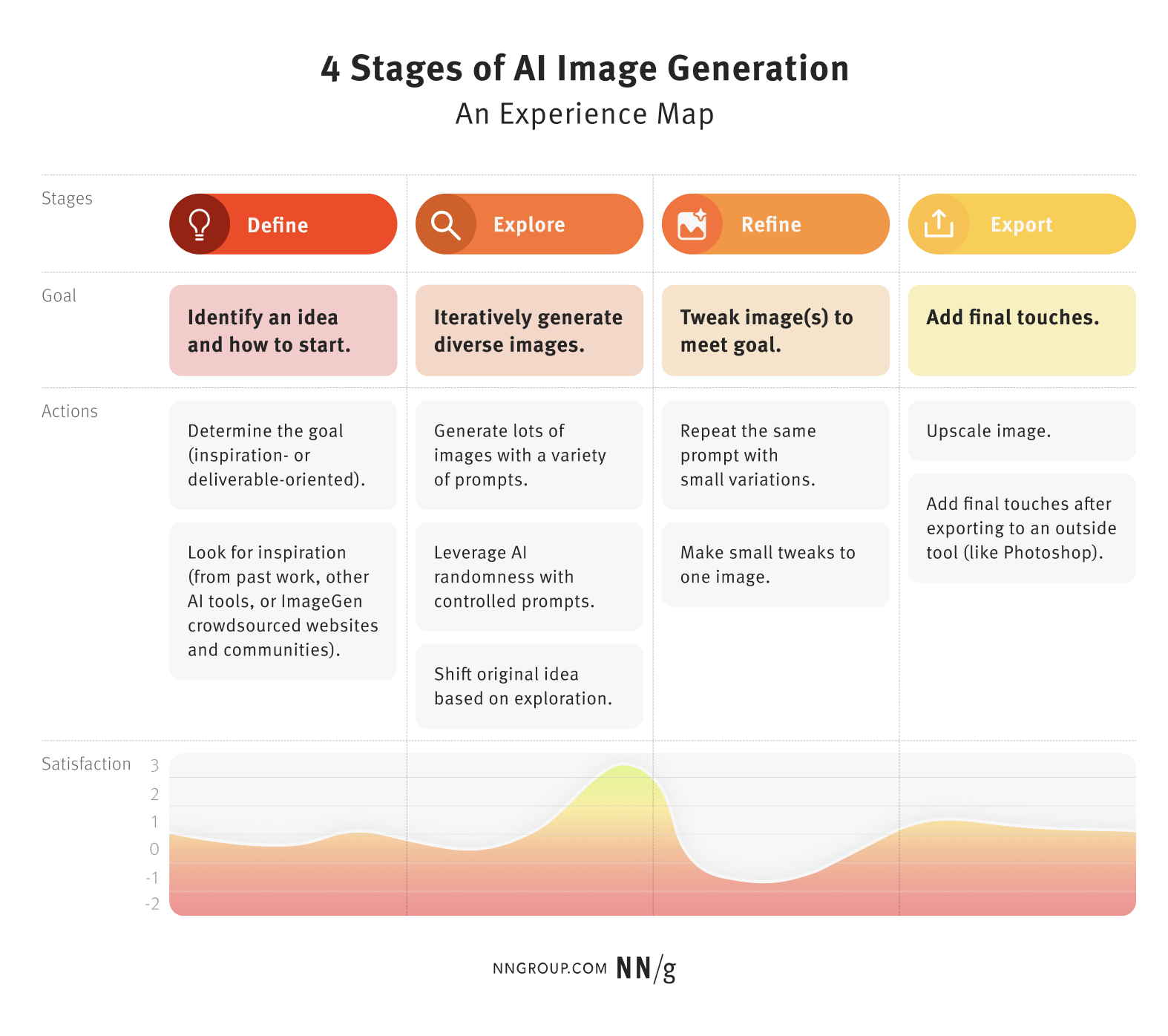
Stage 1: Define
Users start the image-generation process by defining their goal and then thinking about how to achieve it.
Determining the Goal
Users’ goals were either inspiration-oriented or deliverable-oriented.
Inspiration-Oriented
People used image-generation tools to gather inspiration when they lacked a predetermined idea for their final output, seeking concepts rather than specific details or export-ready images.
Examples from our study included:
- gathering inspiration for Second-Life furniture
- creating a catalog of possible designs for anthropomorphic animals
- exploring logo concepts for a client
Deliverable-Oriented
Other users had the primary goal of creating a polished, high-fidelity output, striving for perfection with minimal need for adjustments outside of the AI tool. These images serve as final products, requiring attention to detail and iteration.
Examples from our study included:
- generating final visuals for a board game
- designing final client posters
- crafting storyboards for a movie
Determining How to Achieve the Goal
Once users define their goals (whether inspiration-oriented or deliverable-oriented), they think about how to achieve it.
This step felt overwhelming to some study participants due to the articulation barrier. Users overcame this blank-page problem by referencing other sources, including past images, instructions from generative-AI (genAI) chatbots, or external resources.
Past Images as a Starting Point
Some participants relied on scrolling through images they had generated previously, looking for something similar to their current goal.
Because many participants had worked on several projects like the one they undertook during our sessions, they had a massive bank of past examples to choose from when starting a new task.
Generative-AI Chatbots as a Starting Point
Many of our participants regularly used chatbots, mostly ChatGPT, as a source of image-generation prompt ideas at the beginning of their creative process.
One of them, who attempted to create posters for a children’s bedroom, asked ChatGPT for 10 ideas for Lego vehicle toys he could display on the posters.
Another participant wanted to explore industrial-design concept art. He prompted ChatGPT to give him 5 examples of new futuristic materials, which he then used in the prompt to create the concept art.
Participants also used genAI chatbots to generate prompts for their ideas, then copied and pasted these prompts into Midjourney.
External Resources as a Starting Point
Some study participants visited websites like Midlibrary, a crowdsourced repository of AI-generated images and prompts to pick ideas from.
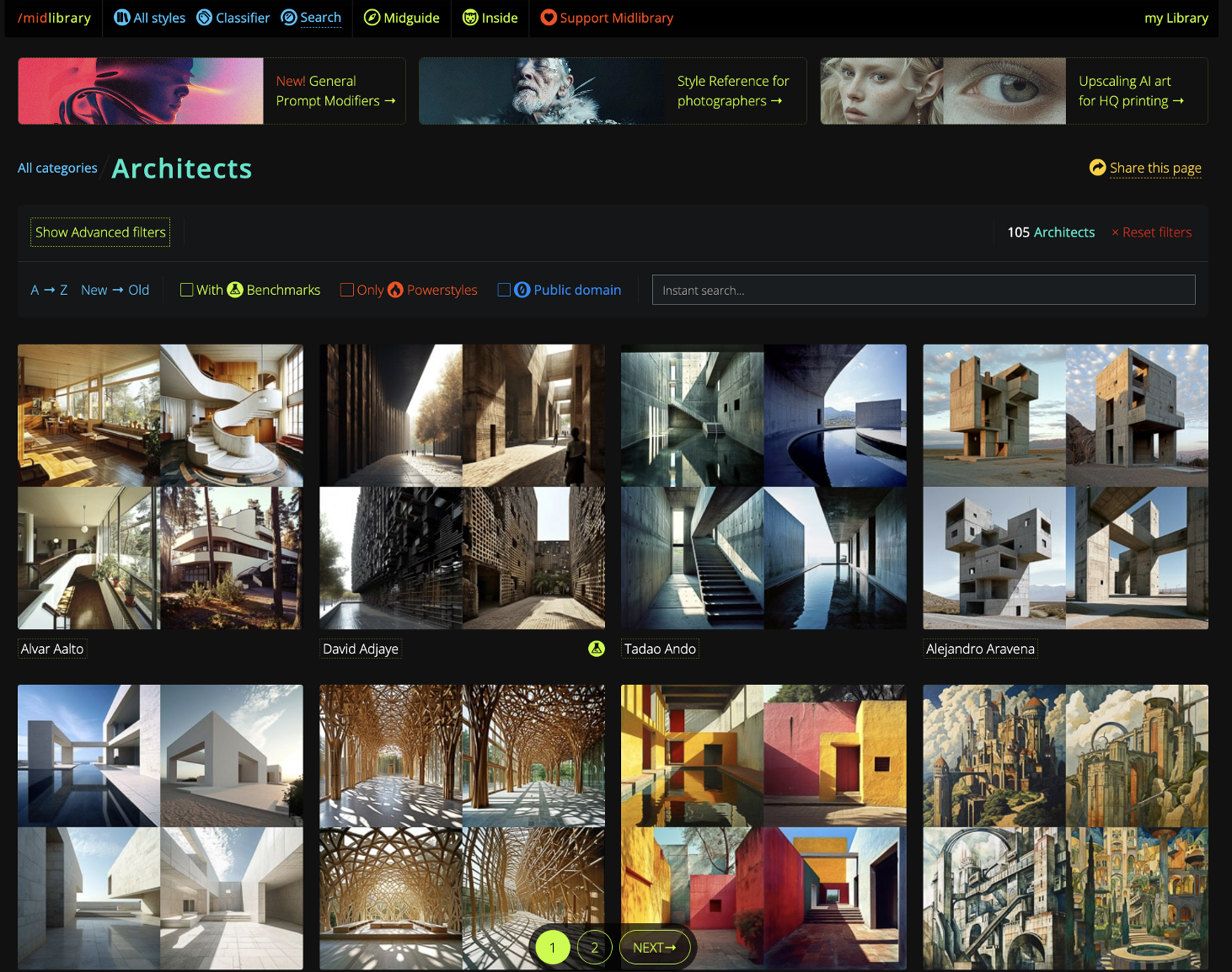
Stage 2: Explore
The output of the first stage is an articulated idea, vision, or prompt for an image. In stage 2, users attempt to create an image that broadly matches their vision; this image can then be tweaked to reach the final output.
Creating Quantity
After defining their goal, participants used the tool to generate images. The exploration phase typically involved creating many images (anywhere from 20 to 80 images), to increase the likelihood that one image aligns with their vision enough.
For example, one of our participants wanted to create an image of a Lego-inspired toy tractor. He generated 20 batches of images in Midjourney (for a total of 80 images) and selected one image. Another participant wanted to generate an image of a steampunk-themed sofa. They created several prompts for the same concept and tested all of them.
The expert users we observed employed two common strategies to create quantity efficiently: prompt repetition and prompt variation.
Prompt Repetition
This technique involves repeating the same prompt to “exhaust the possibilities” related to one creative direction. Due to the inherent randomness of the AI image-generation algorithm, this practice resulted in many variations of the same concept, allowing people to choose what they liked best.
Expert users in our study used prompt shortcuts like the –r 10 suffix in Midjourney to quickly generate many (in this case 10) batches of images based on the same prompt.
Prompt Variation
Prompt variation involves writing several slightly different prompts for the same target image. Participants with large prompt vocabularies used prompt variation to explore multiple creative directions.
For example, one participant used prompt variation to generate an anthropomorphic fox character:
Prompt 1: anthropomorphic fox, cowboy shot, highly detailed
Prompt 2: anthropomorphic fox human hybrid, full body mascot, highly detailed
Prompt 3: fox head on a human
This prompting strategy came with challenges. Coming up with numerous prompt alternatives rapidly is cognitively challenging for regular users.
Expert users, however, have a better technical vocabulary: they know that terms like “turnaround sheet” will create many character variations or use built-in functions like the /describe feature in Midjourney (which provides 5 different prompt phrasings for a given image). They also use model prompts from sources like Midlibrary and are generally better at figuring out how to overcome the articulation barrier.
Discovering New Ideas During Exploration
While users often establish an initial vision during the Define stage, the Explore phase frequently uncovers ideas that surpass their original concepts. It was typical for users to abandon their initial idea in favor of an image better aligned with their vision or preferences.
According to the participants, AI-generated ideas often came as “a pleasant surprise,” leading them to explore new and improved directions. For many, discovering alternatives superior to their original vision was the major benefit of AI image generation.
Once users have created an image that loosely matches their expectations regarding subject, aesthetics, and composition, they tend to move on to the next step, Refine, to address imperfections and further improve the image.
However, in inspiration-oriented instances, where details are either unimportant or will be refined using tools external to the AI image-generation tool, the Explore stage could mark the end of the AI image-generation process.
Stage 3: Refine
During this stage, users tweak the image to get it as close to the desired output as possible. This stage is the most complex and difficult, even for experts. One participant using Midjourney said, “Midjourney is a generator, not an [image[ editor.” Users refine images in many ways. We observed participants add, remove, or change specific objects from images or change the composition to achieve the final result.
Lack of User Control: Fighting the AI
One study participant wanted to create an image of a steampunk-themed sofa. After multiple iterations, she got an image of a sofa she was happy with but wanted to change the upholstery from plain to striped. This edit proved difficult, as the appearance of the sofa would change every time she tried to make an adjustment, due to the randomness of the AI tool.
Changing small details of an AI-generated image can be an arduous, time-consuming task. Participants often experience frustration due to the lack of user control (usability heuristic #3) inherent to current AI image-generation tools. Unlike traditional image-processing software, these tools offer limited support for fine adjustments, which means users end up fighting against the AI to achieve desired outcomes. Consequently, users frequently end up dissatisfied as the final images often fall short of perfection.
Stage 4: Export
After reaching the limit of what they can achieve through prompting, users export the image out of the tool.
They add finishing touches and final edits using other tools like Photoshop, upscale the image (i.e., increase the image size) using an AI upscaler, or add text overlaying the image. This phase concludes their design process for that specific task.
Nonlinear Variations
While we’ve described four stages in the AI image-generation process, not everybody will go through all of them. Individual users’ goal and experience ultimately determines how their journey looks and how much time they spend in each stage.
Inspiration-Oriented Creators Spend More Time Exploring
Users with an inspiration-oriented goal spend more time in the Define and Explore stages and may skip the Refine and Export stages since they want only image ideas rather than image deliverables.
For example, one participant looked for inspiration for a logo. He created a few different logo ideas in Midjourney but decided to stop there because, after gathering potential design directions, he planned to make the final logo in Figma.
Deliverable-Oriented Creators Spend More Time Refining
When the AI-generated image was used as the final deliverable, users attempted to get the details as close to perfect as possible. They started with the Define and Explore stages but then spent most of their time in the Refine and Export stages. The lack of user control in the Refine stage made this process challenging and even frustrating for users.
For example, the participant creating the poster of a Lego-inspired tractor for a kid’s bedroom used ChatGPT to come up with the idea and then spent most of the time in Midjourney, transforming that idea into a polished final image.
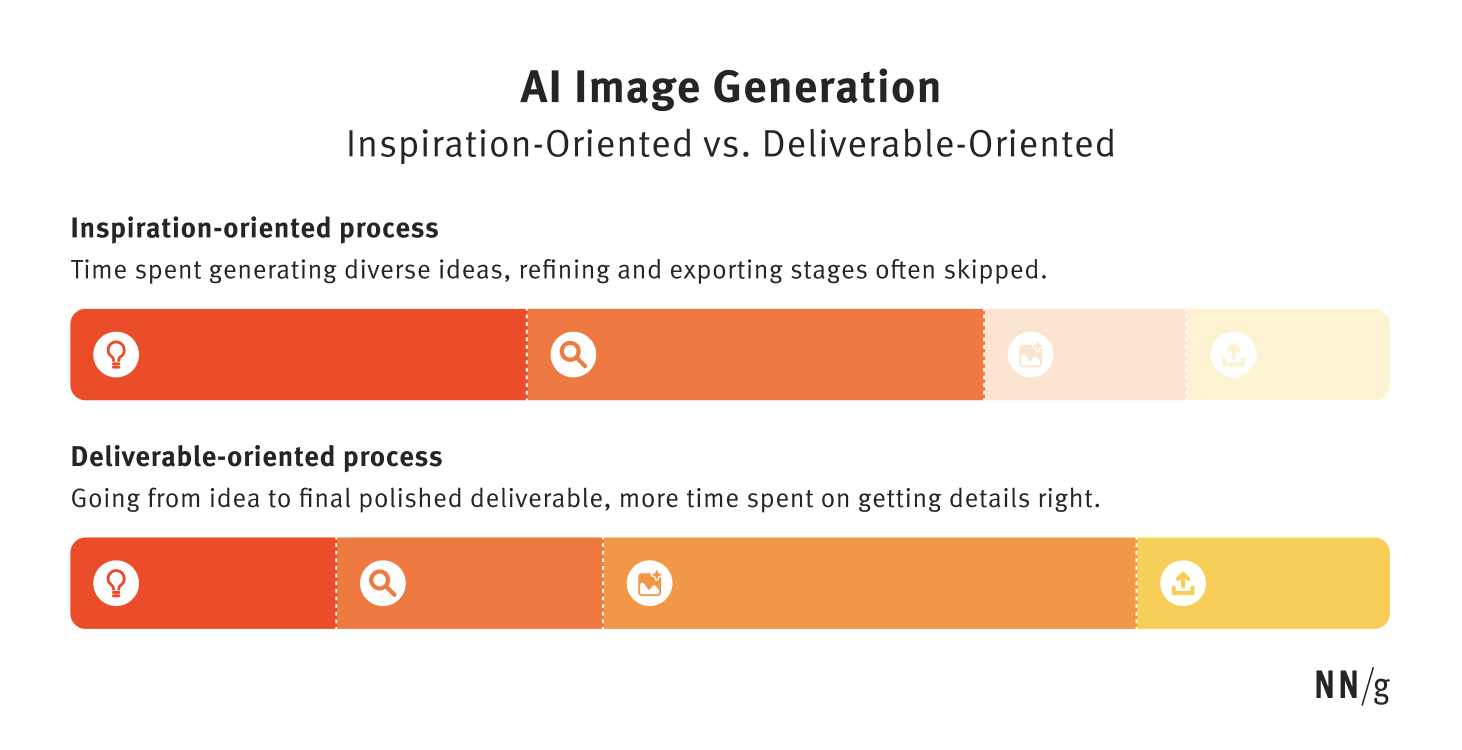
Conclusion
Understanding experts’ image-generation process is valuable for both users and designers of AI image-generation tools.
Users of AI image-generation tools can learn how to become better at achieving their goals. For example, they can use chatbots to create their prompts and can start vary their prompts as they iterate.
Understanding users’ creative processes helps designers of AI image-generation products to identify pain points and areas for improvement (like the need to give users more control during the Refine stage). By recognizing the different needs of inspiration-oriented and deliverable-oriented users, designers can create more tailored and efficient tools, that not only facilitate creative exploration but also streamline workflows, increasing the likelihood users achieve their goals.
Source: https://www.nngroup.com/articles/ai-imagegen-stages/